ICDCN
2023
24th International Conference On
Distributed Computing And Networking
4-7th January, 2023
|
Department of Computer Science and Engineering, IIT Kharagpur
Keynote Speakers
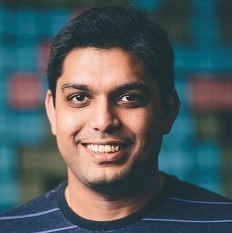
Ranveer Chandra
Microsoft Research
Managing Director, Research for Industry
Partner Manager, Networking Research
CTO, Agri-Food
Bio:
Ranveer Chandra is the Managing Director for Research for Industry, and the CTO of Agri-Food at Microsoft. He also leads the Networking Research Group at Microsoft Research, Redmond. Previously, Ranveer was the Chief Scientist of Microsoft Azure Global. His research has shipped as part of multiple Microsoft products, including VirtualWiFi in Windows 7 onwards, low power Wi-Fi in Windows 8, Energy Profiler in Visual Studio, Software Defined Batteries in Windows 10, and the Wireless Controller Protocol in XBOX One. His research also led to a new product, called Azure FarmBeats. Ranveer is active in the networking and systems research community, and has served as the Program Committee Chair of IEEE DySPAN 2012, and ACM MobiCom 2013.
Ranveer has published more than 100 papers, and holds over 150 patents granted by the USPTO. His research has been cited by the popular press, such as the Economist, MIT Technology Review, BBC, Scientific American, New York Times, WSJ, among others. He is a Fellow of the IEEE, and has won several awards, including best paper awards at ACM CoNext 2008, ACM SIGCOMM 2009, IEEE RTSS 2014, USENIX ATC 2015, Runtime Verification 2016 (RV’16), ACM COMPASS 2019, and ACM MobiCom 2019, the Microsoft Research Graduate Fellowship, the Microsoft Gold Star Award, the MIT Technology Review’s Top Innovators Under 35, TR35 (2010) and Fellow in Communications, World Technology Network (2012). He was recently recognized by the Newsweek magazine as America’s 50 most Disruptive Innovators (2021). Ranveer has an undergraduate degree from IIT Kharagpur, India and a PhD from Cornell University.
Talk Title:
Empowering Farmers With Affordable Digital Agricultural Solutions
Talk Abstract:
Data-driven techniques help boost agricultural productivity by increasing yields, reducing losses and cutting down input costs. However, these techniques have seen sparse adoption owing to high costs of manual data collection and limited connectivity solutions. In this talk we will describe our innovations that leverage the Internet of Things and Artificial Intelligence to help make affordable digital agriculture solutions. We will also present our product based on this research, which is in preview, and can be used by partners to build their digital agriculture solutions.
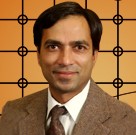
Vijay K Garg
Professor, Electrical and Computer Engineering Department
Director, Parallel and Distributed Systems Laboratory, University of Texas, Austin.
Bio:
Vijay Garg is a Cullen Trust Endowed Professor in the Department of Electrical & Computer Engineering and Department of Computer Sciences at The University of Texas at Austin. He is an IEEE Fellow and is the director of the Parallel and Distributed Systems laboratory at UT Austin. His research contributions are in the areas of distributed algorithms, global predicate detection, distributed debugging and simulation, fault-tolerance, lattice theory and supervisory control of discrete event systems. His research has been supported by NSF, IBM, Texas Advanced Research Program, TRW, SRC, and Compaq among others. He has received Lepley Teaching Award (ECE Departmental Teaching Award, 2015), Departmental nomination for Lockheed Martin Aeronautics Company Award for Excellence in Engineering Teaching (2015), UT Outstanding Inventor (2011), Best Paper Award at 17th International Conference on Runtime Verification (RV'2017), Best Paper Award at 12th SSS (2010) etc.
Talk Title:
Lattice Linear Predicate Algorithms For The Constrained Stable Marriage Problem With Ties
Talk Abstract:
We apply the Lattice-Linear Predicate Detection Technique to derive parallel and distributed algorithms for various variants of the stable matching problem. These problems are: (a) the constrained stable marriage problem, (b) the super stable marriage problem in presence of ties, and (c) the strongly stable marriage in presence of ties. All these problems are solved using the Lattice-Linear Predicate (LLP) algorithm showing its generality. The constrained stable marriage problem is a variant of the stable marriage problem in the presence of lattice-linear constraints such as ``Peter's regret is less than that of Paul.'' For the constrained stable marriage problem, we present a distributed algorithm that takes $O(n^2)$ messages each of size $O(\log n)$ where $n$ is the number of men in the problem. Our algorithm is completely asynchronous. Our algorithms for the stable marriage problem with ties are also parallel with no synchronization.
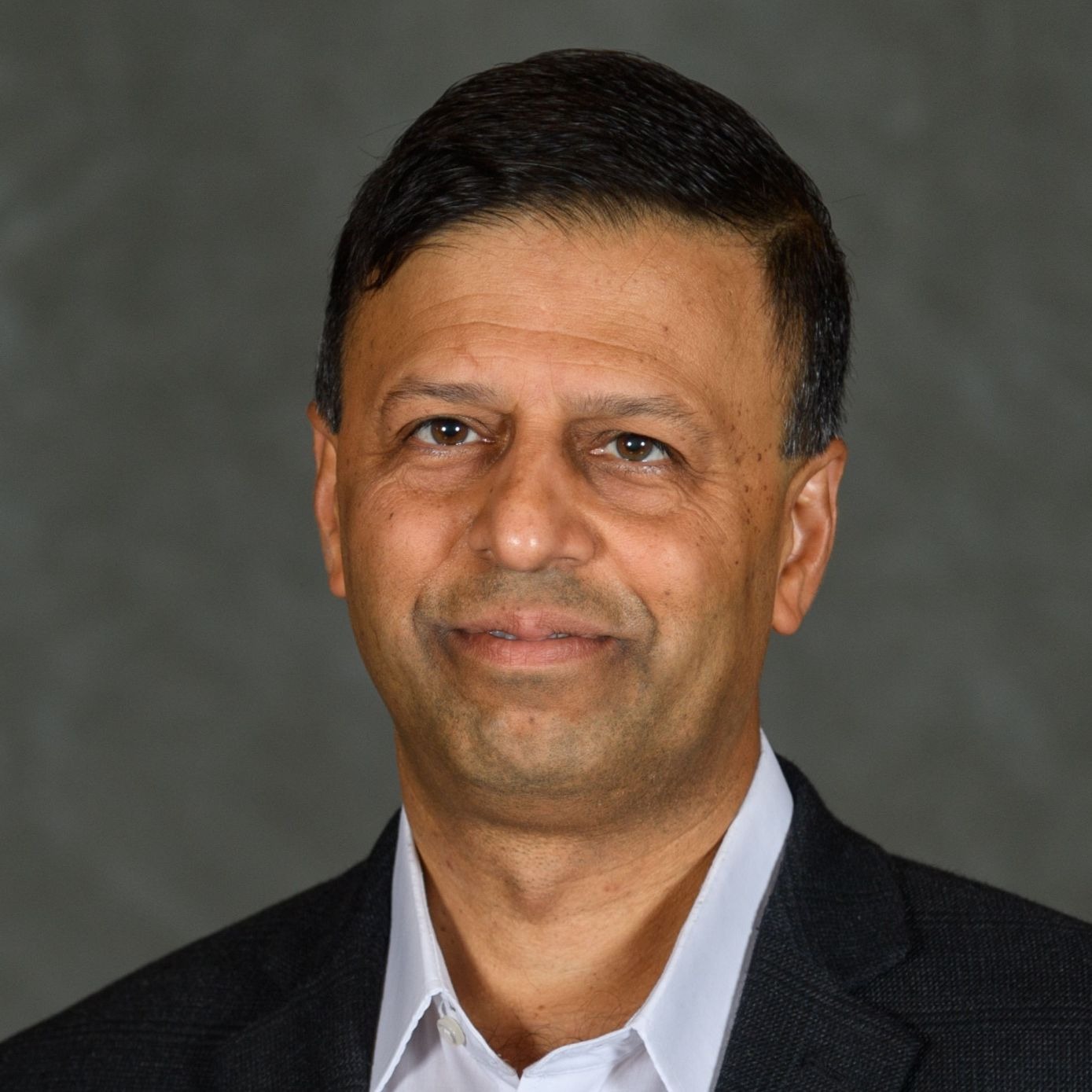
Nitin H Vaidya
Chair of Computer Science
Georgetown University
Bio:
Nitin Vaidya is the Robert L. McDevitt, K.S.G., K.C.H.S. and Catherine H. McDevitt L.C.H.S. Chair of Computer Science at Georgetown University. He received Ph.D. from the University of Massachusetts at Amherst. He previously served as a Professor and Associate Head in Electrical and Computer Engineering at the University of Illinois at Urbana-Champaign. He has co-authored papers that received awards at several conferences, including 2015 SSS, 2007 ACM MobiHoc and 1998 ACM MobiCom. He is a fellow of the IEEE. He has served as the Chair of the Steering Committee for the ACM PODC conference, as the Editor-in-Chief for the IEEE Transactions on Mobile Computing, and as the Editor-in-Chief for ACM SIGMOBILE publication MC2R.
Talk Title:
Security and Privacy for Distributed Optimization and Learning
Talk Abstract:
Consider a network of agents wherein each agent has a private cost function. In the context of distributed machine learning, the private cost function of an agent may represent the “loss function” corresponding to the agent’s local data. The objective here is to identify parameters that minimize the total cost over all the agents. In machine learning for classification, the cost function is designed such that minimizing the cost function should result model parameters that achieve higher accuracy of classification. Similar problems arise in the context of other applications as well, including swarm robotics. Our work addresses privacy and security of distributed optimization with applications to machine learning. In privacy-preserving machine learning, the goal is to optimize the model parameters correctly while preserving the privacy of each agent’s local data. Privacy-preserving machine learning is becoming important due to the increasing reliance on user-generated data for machine learning. In security, the goal is to identify the model parameters correctly while tolerating adversarial agents that may be supplying incorrect information. When a large number of agents participate in distributed optimization, security compromise of some of the agents becomes increasingly likely. We constructively show that such privacy-preserving and secure algorithms for distributed optimization exist. The talk will provide intuition behind the design and correctness of the algorithms.
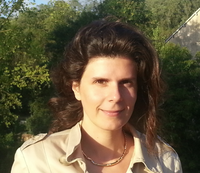
Niki Trigoni
Professor of Computing Science, Oxford University, UK
Governing Body Fellow, Kellogg College
Bio:
Niki Trigoni is a Professor at the Oxford University Department of Computer Science and a fellow of Kellogg College. She obtained her DPhil at the University of Cambridge (2001), became a postdoctoral researcher at Cornell University (2002-2004), and a Lecturer at Birkbeck College (2004-2007). At Oxford, she is currently Director of the EPSRC Centre for Doctoral Training on Autonomous Intelligent Machines and Systems, a program that combines machine learning, robotics, sensor systems and verification/control. She also leads the Cyber Physical Systems Group, which is focusing on intelligent and autonomous sensor systems with applications in positioning, healthcare, environmental monitoring and smart cities. The group’s research ranges from novel sensor modalities and low level signal processing to high level inference and learning.
Talk Title:
Lifting The Veil Over Emergency Scene Understanding
Talk Abstract:
Scene understanding algorithms allow machines to grasp the content and meaning of ambient scenes and even infer aspects that are not directly observable. Combined advances in sensing, robotics and machine learning have recently led to scene understanding algorithms that have transformed smart city applications, like transport, healthcare and energy. However this technology has found slower uptake in the challenging world of blue light emergency response. In this talk I will present some of the key challenges faced by intelligent sensor systems in emergency situations including dynamic changes of the environment, lack of preinstalled network or sensor infrastructure, and operation in previously unseen and unpredictable situations. I will then present recent approaches to addressing these challenges including multi-modal sensing, cross modality training and lightweight approaches to human-robot interaction.
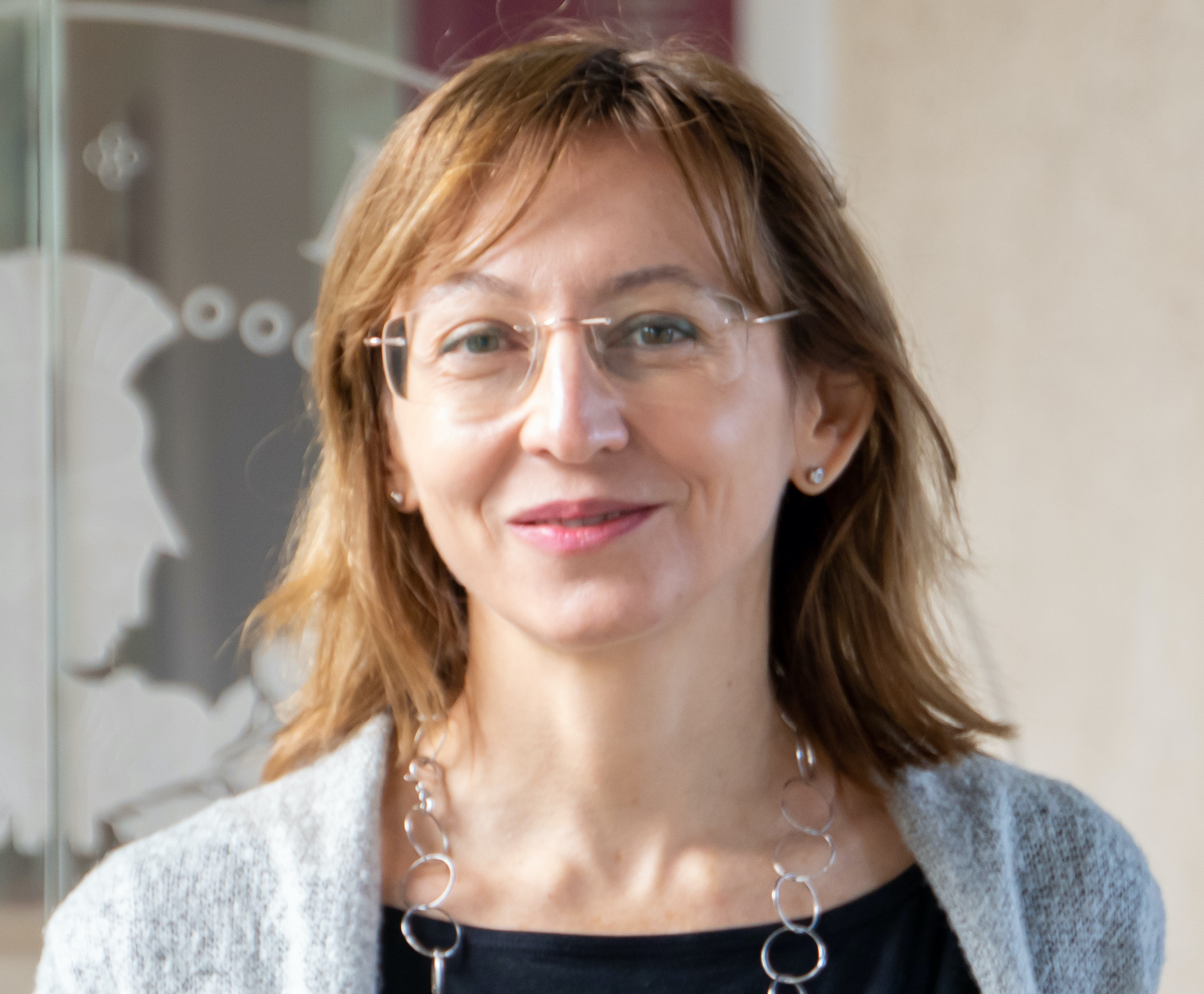